As in every area of the economy, digital transformation has disrupted project management. In order to manage projects professionally, we do not need paper documents anymore. We do not need to work from specific locations, neither. Project data, essential for business management in the Project Economy, is growing exponentially. More and more people collaborate on projects, sharing more and more technical and management data, and organizations are executing more and more projects. With so much project management data available, could algorithms manage projects automatically? What will be our role as project management professionals?
In this first article on artificial intelligence, we will review the meaning, history, and potential of artificial intelligence. The next article will cover artificial intelligence applications to manage projects, programs, and portfolios professionally.
Artificial Intelligence (AI) is the intelligence demonstrated by machines, as opposed to the natural intelligence displayed by humans or animals. John McCarthy coined the term of “artificial intelligence” in the summer of 1956 at the Dartmouth Workshop, held at Dartmouth College in Hanover, New Hampshire, USA. The big expectations coming from AI materialized with large founding for research programs. Artificial Intelligence lived its “golden age” during the following 20 years, getting great achievements with algorithms on searching and game optimization, expert systems, and the initiation of Natural Language Processing (NLP).
In the mid-1970s, most AI research programs were canceled as expectations were not met. AI entered the so called “AI winter”. Expectations such as “AI being comparable to human intelligence in late 70s” were technically undoable back then, given the limited computer capacity on processing and storage.
During the decade of 1980, there were great improvements on a kind of algorithms named artificial neural networks. An artificial neural network algorithm follows a machine learning model inspired on the biological neural networks that constitute animal brains. The network can learn relationships between input and output data. Once it has been trained, the data learned and stored as parameters in the hidden layers make it possible to generalize further knowledge beyond the examples given.
For a brief introduction, you can watch this video on the fundamentals of artificial neural networks:
In the 90s, AI resurfaced again. Expectations were narrowed this time over specific tasks aimed to expert systems and neural networks. In 1997, IBM supercomputer DeepBlue defeated chess world champion Garri Kasparov. Big money came to fund many expert systems in the area of manufacturing.
AI entered its maturity phase in the 2010 decade. In 2016, software AlphaGo, by Google DeepMind, beat the world champion at Go, which is considered as the most complex game.
Researchers, software engineers, companies and academy institutions began to trust finally on the promising future of AI, not fearing any new “AI winter”, mainly due to three reasons:
- Computer processing power increased exponentially, following Moore’s law. Precisely, AI engineers started using video cards, whose architecture is optimized for matrix calculation, the type of intensive computational work needed by artificial neural network algorithms.
- The effectiveness and continuous improvement of artificial neural network algorithms like generative adversary neural networks.
- Huge amounts of data available on the internet to train artificial neural network algorithms. If the right curated data sets are used, then AI overcomes humans by far, when it comes to recognizing patterns out of non-structured, complex, too large amount of data, a.k.a. Big Data.
Nowadays, in the 2020 decade, AI applications for image recognition and Natural Language Processing (NLP) are increasingly becoming a commodity. AI applications based on generative models are marketed to create new images and text. Language processing is advancing by leaps and bounds on virtual assistants and chatbots, and now most applications are capable of passing the conversational Turing test.
Internet of Things (IoT) connects more and more devices on the internet, and it will soon connect more and more robots. Factories are introducing autonomous robots in operations. Autonomous cars will get full automation level soon.
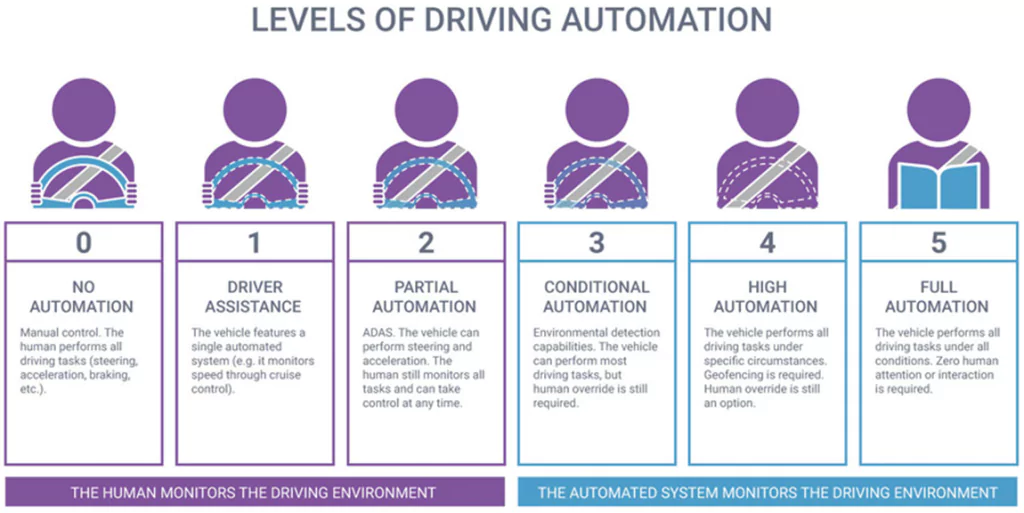
All of the above are applications of what is called narrow AI. There are many years ahead before we can talk about Artificial General Intelligence. There are three types of Artificial Intelligence:
- Artificial Narrow Intelligence (ANI) refers to AI that handles one task. All the AI methods we use today fall under narrow AI.
- Artificial General Intelligence (AGI) refers to a machine that can handle any intellectual task. AGI is an intelligence at the same level of a human being, with the ability to do the same knowledge activities than a person. The ideal of AGI has been all but abandoned by the AI researchers because of lack of progress towards it in more than 50 years despite all the effort.
- Artificial Super Intelligence (ASI): Nick Bostrom defines superintelligence as «any intellect that greatly exceeds the cognitive performance of humans in virtually all domains of interest».
A related dichotomy is “strong” and “weak” AI. Strong AI would amount to a mind that is genuinely intelligent and self-conscious. Weak AI is what we actually have, namely systems that exhibit intelligent behaviors despite being mere computers.
AI is already a ubiquitous, exponentially growing reality. As individuals, every day, we all use smart assistants, browsers, search engines, social networks, etc. All of us receive personalized advertising and content recommendations continuously. At a business level, AI is the ground of the 4th Industrial Revolution.
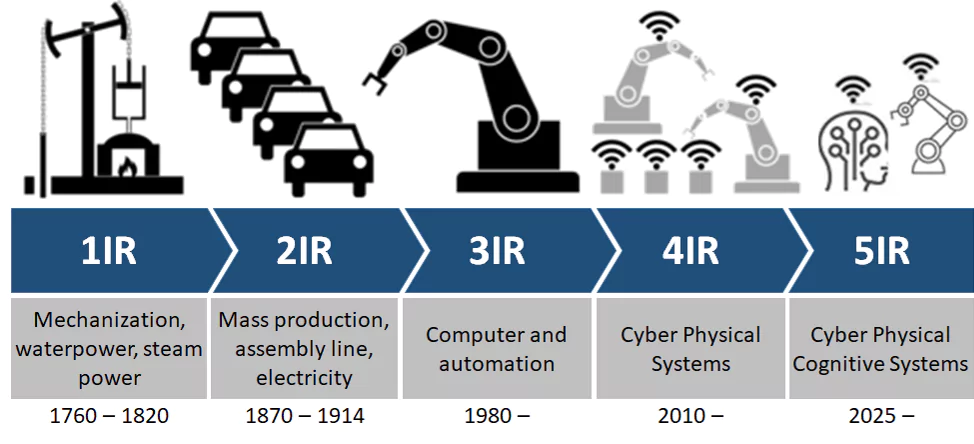
In the next article, we will talk about how artificial intelligence could be applied to manage projects, programs, and portfolios. In the next article, we will talk about how artificial intelligence could be applied to manage projects, programs, and portfolios. Project managers surely are not to be replaced by AI, but they would better know how to use it in their day-to-day practice.
Jose Barato
Related posts
Categories
- Business (16)
- Demand Management Roles (14)
- Frequently Asked Questions (7)
- Guide (26)
- People (23)
- Assignments (2)
- Feedback (2)
- Project Team (3)
- Tracking Time And Expenses (2)
- Process (9)
- Closing (2)
- Executing And Controlling (2)
- Planning (1)
- Project Management (67)
- Management Frameworks (18)
- Organization Owner (OO) (3)
- Project Economy (54)
- Tools (19)
- Supply Management Roles (5)
- Training (6)
- Uncategorized (1)